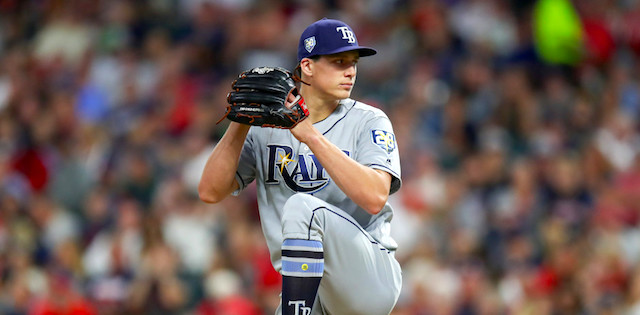
Over the last 10 or so years, baseball fans have seen all kinds of new statistics pop in front of their faces. Some people really like these new metrics, and some people really do not. Judging by the fact that you are reading this article, I am guessing that you fall into that first grouping.
Just because a statistic is available to the masses does not mean that there is not an advantage to be had from the access. A statistic is only as good as the context and application a decision-maker uses alongside it.
For that reason, I want to dive into the true value of the "hard-hit rate" statistic when applied to fantasy analysis.
Featured Promo: For this week only, take 50% off any full-season or yearly Premium Pass on the site! Just enter discount code THANKS when checking out. Thanks for being a reader, and Happy Holidays! Sign Up Now!
Different Strokes
This metric comes in different varieties. FanGraphs has a hard-hit rate metric for every player in every season since the 2002 MLB season. More recently we have seen statistics like average exit velocity and barrel rate become more prominent. They all do the same thing in their own way - try to assess the quality of contact being made by a hitter. This is a noble goal, of course. Not all fly outs are made equal!
You would much rather invest in a guy that has just gone hitless in 10 at-bats with seven balls absolutely smoked right at the left fielder rather than a guy who blooped a handful of doubles down the line without actually hitting any balls well. However, if we put too much emphasis on a statistic without really understanding its predictive power, we get ourselves into trouble.
My inspiration for this post came from the reigning American League Cy Young Award winner, Shane Bieber. The Indians ace had fantastic numbers in the 2019 season after being a very late draft pick in fantasy leagues, proving to be one of the most valuable fantasy players of the season.
Despite that, there was some hesitation on Bieber heading into the 2020 season because of the alarming batted ball numbers:
Most people reading this know that a 43% hard-hit rate is high, but it is always worth adding on further context. Taking a look at the last seven MLB seasons and looking at pitchers that threw 50 or more innings, here is the distribution of hard-hit rates posted in that time frame:
The average rate there is 33%, with Bieber's 43% falling in the top 10% of outcomes. The xWOBACON (expected weighted on-base average on contact, essentially just another metric that tries to show how well hitters hit the ball when they made contact) lined up similarly when compared with the rest of the league. These were notable numbers. Common sense would suggest that a pitcher that gives up this many well-struck balls but still posted elite ERA and WHIP numbers would be likely to see lots of regression, right? Right???
Certainly, the Biebs had a healthy amount of good fortune that turned a lot of should-be extra-base hits into fly-outs! Well, not so fast. The beautiful thing about baseball is that we almost always have enough data to back-test our ideas.
Targeted Anecdotes
First, I took another unscientific look at the data. By unscientific, I mean I just picked a small number of pitchers at random and plotted their hard-hit rates over the 2015-2020 sample.
It is not really fair to call this group of pitchers "randomly" selected. Clearly, I just picked four pitchers that have had Hall of Fame level careers that are still near the top of their game, as well as two guys that are pretty well known for being mediocre to flat-out horrible.
Knowing that the standard deviation(a measure of the spread of a distribution of numbers) is 5.4, is important here. So if you have an average hard-hit rate one year (33%), and then see a jump to 44% the next season, that is a two standard deviation jump - which would be really unexpected with a statistic that is normally distributed (and therefore predictable). What do we see here? Randomness.
Take Kershaw for example. The Dodgers' ace kept things pretty steady from 2015-2017, with a hard-hit rate between 25% and 29%. Then he saw a two standard deviation jump to 36% in 2018, followed by another standard deviation jump to 42% in 2019, before bringing things right back to the average level of 33% last season, a huge decline of 9%. You see these jumps for every pitcher visualized here, there is very little consistency pictured.
Only seven different pitchers have been mentioned thus far in this post, and that is no way to make a sound statistical argument. A deeper investigation is required.
Correlation
Our goal here is to find out whether a pitcher's hard-hit rate one year has any predictive power over next year. If we find that it does, we want to take high hard-hit rates from the previous season pretty seriously, and vice versa.
I did some Python coding on the MLB pitching stats from the years 2016-2019. I took these years in pairs (so, first I looked at 2016 and 2017, then 2017 and 2018, then 2018 and 2019), and found every pitcher that had at least 50 innings thrown in each pairing. After that, I found their hard-hit rate in year one, added it to a list, and then found their rate in the year after that, and added it to the same location in another list.
So for example, Aroldis Chapman had a 28.1% hard-hit rate in 2016 and a 27.4% in 2017. Those two numbers were compared to each other when I ran the correlation check. Correlation only works with long lists, and using this methodology of comparing season N with season N+1 for every qualified pitcher a list nearly 700 numbers in length. I did this for a variety of statistics and found the correlation coefficients for the resulting lists.
Generally speaking, anything over 0.3 shows a positive correlation, with that correlation getting stronger as you approach one, which is a perfect correlation. An example of this would be: the longer you exercise, the more calories you burn. Those two variables (time spent and calories burned) are highly correlated - one directly affects the other. As the correlation coefficient gets closer to one, the stronger the relationship is. Anything between 0.3 and 0.5 is a pretty weak correlation, and therefore not very useful for making predictions. Anything over 0.7 or so will be a reliable indicator for prediction.
Here are the correlation coefficients for all of the stats I checked. The last row shows the result when using long lists of completely random numbers - the purpose of that being to show what true randomness looks like in the correlation world.
Ground ball rate and strikeout rate are the true standouts. If a pitcher has a high groundball rate (over a significant sample of innings, remember that this sample was only for pitchers reaching 50 innings) one year, you can feel very confident that they will do the same the next year. Same with strikeouts. These metrics are typically pretty steady from year-to-year at the individual pitcher level.
You see that hard-hit rate came in pretty low, but still shows signs of weak positive correlation. While you cannot call it "random," this coefficient really does not inspire much confidence in the predictive power of the metric. If you see a pitcher give up a high hard-hit rate in 2019, there is no real reason to expect them to do the same the following year.
I also went ahead and checked how hard-hit rate correlates with other statistics. Using all of the data from 2015-2019, I checked how each hard-hit rate correlated with other relevant fantasy pitching categories like ERA, WHIP, and HR/9. The only stat showing any correlation above randomness was HR/9, and it was a weak correlation coefficient of 0.44. Even with something that makes that much logical sense (hard-hit balls turning into home runs), there was not really a significant relationship.
Application
While this was more of a study to test a theory, there is certainly some resulting application that we can use for the 2021 season. The application of that would namely be to go see which pitchers gave up high hard-hit rates in 2020, and then keep them in mind when ADP data starts rolling out. There is a chance that fantasy players get a bit scared off of these pitchers because of these high hard-hit rates and their draft stock falls because of it. From what this analysis shows, there is not a strong reason to downgrade a pitcher because he gave up a lot of hard-contact the year before. There is not enough predictive power of the statistic to justify it.
To give you some names, here is a leaderboard based on the 2020 hard-hit rates and comparing them to pitcher's averages from the previous five seasons:
Note that I am not saying that you should expect McCullers to come back to his previous average of 29% hard-hit rate or anything like that. That would be nonsense based on everything I just said. There is no way to predict this, but these are the pitchers that might fall in drafts because fantasy players are seeing these "alarming" batted ball metrics from 2020 and downgrading guys when they should not be.
It is even better when you see pitchers like McCullers and Glasnow, who get a ton of strikeouts, at the top of the list. Strikeout rate goes hand-in-hand with hard-hit rate in a lot of ways just because a high strikeout pitcher is hurt less by a high hard contact rate because they were not allowing as many balls in play in the first place. Think of it like this:
Glasnow faces 100 batters, strikes out 30 of them (a 30% strikeout rate), but gives up a high 40% hard-hit rate. Let's just say he walked nobody for the sake of easier math. That 40% only applies to the 70% of plate appearances that were left after we take out strikeouts. So that 40% rate equates to 28 well-struck balls (100 * 0.7 * 0.4). For a pitcher with a 20% strikeout rate, that same 40% rate equates to 32 hard-hit balls (100 * 0.8 * 0.4), four more balls that could have done damage to his stat line. High strikeout pitchers coming off a year where they saw a spike in hard-hit balls against is the sweet spot here. This is exactly where Bieber lined up heading into 2020, and you all saw how that turned out.
Too Long, Didn't Read Summary
Hard-hit rate is largely random, and you should not downgrade a pitcher because they gave up a lot of hard-contact the year prior. If your league mates do this, you will have an advantage in finding value at the starting pitcher position.
Download Our Free News & Alerts Mobile App
Like what you see? Download our updated fantasy baseball app for iPhone and Android with 24x7 player news, injury alerts, sleepers, prospects & more. All free!
More 2021 Fantasy Baseball Advice