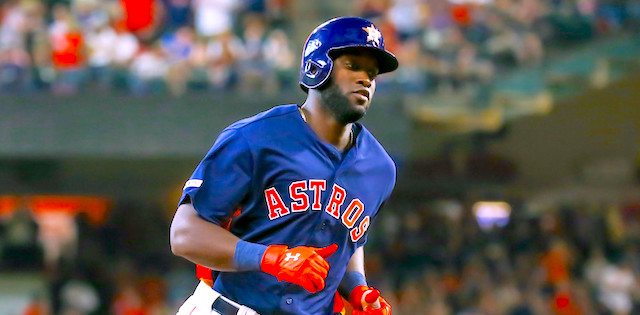
It can be fun to look back at previous things you’ve written, gaining a window into your state of mind in that moment. I originally wrote much of this piece back in March, when baseball seemed like a distant dream. From March:
"The last few weeks have been challenging. In the grand scheme of things, our collective safety and well-being is the only thing that matters. It’s up to each of us to do everything in our power to minimize the impact of COVID-19, like staying home and informed, when possible. In doing so, perhaps there’s still a role for temporary pockets of reprieve — listening, reading, writing, among other things. Personally, I’ve found new podcasts from all the baseball personalities we know and love to be oddly therapeutic — more so than normal. I’d like to think that the two — our civic responsibilities and need for occasional “diversions” — aren’t mutually exclusive. With that, I’ve continued to occasionally draft, read, analyze, listen, write, etc. Not because I know there will be a season — I have no clue — but because, well, I need it!"
Now on the verge of return, I’m cautiously optimistic — and excited — about its return! One piece of analysis I’d been toying with all offseason, and that I’m excited to unveil as we head into this 60-game sprint, is the mental weighting of our shiny new Statcast metrics.
Featured Promo: For this week only, take 50% off any full-season or yearly Premium Pass on the site! Just enter discount code THANKS when checking out. Thanks for being a reader, and Happy Holidays! Sign Up Now!
Statcast: Who's The Best?
Scroll through your Fantasy baseball-related Twitter feed and you’re bound to find numerous references to newer statistics like launch angle, exit velocity and barrels. But which ones are the best? And by how much? And how do they perform relative to “old reliable” metrics, like strikeout and walk rate?
Al Melchior and Alex Chamberlain, both of FanGraphs, took an initial pass at some of these questions back in 2018, emphasizing the importance of barrels and flyball distance for power metrics like HR/FB, ISO and Hard Hit Rate.
Building on their work — and armed with an additional season’s worth of data — I set out to test which metrics best predicted future hitter performance, as defined by weighted on-base percentage (wOBA). While not the perfect measure of 5x5 Fantasy goodness, it serves as a useful proxy for a hitter’s Fantasy value. Worst case, even if it didn’t, perhaps we can better understand who the best hitters are — that’s a skill that’s unlikely to go out of style.
An Attempt At Weightings: "POWA"
To do so, I pulled 2015-2019 (five seasons) data from Baseball Savant for all hitters with at least 500 PA (n = 359 season pairs). You can find the full test results here for all of the metrics tested.
Similar to our weighting of various pitching measures to create ACES, I was seeking to understand: which metrics should we use and how to weight them?
After testing which metrics in one season (“season-n”) were most predictive of the following season’s wOBA (“season-n+1”), the following combination and weightings were found to be most predictive — shown broken into two groups here, plate discipline and contact quality:
(For a description of each metric, take a gander at MLB’s Statcast glossary.)
Plate Discipline | |
Strikeouts (K%) | 27% |
Walks (BB%) | 20% |
Total | 47% |
Contact Quality | |
Avg. EV | 15% |
Barrel/BBE % | 14% |
EV95+ % | 10% |
Max EV | 6% |
Poorly Topped% | 5% |
Avg. Distance | 3% |
Total | 53% |
This seems to make sense! A near 50-50 mix of plate discipline (47%) and contact quality (53%) contributed to better future performance — thus, the formula for our new metric is:
- Minimize strikeouts (27%), take walks (20%)
- Hit the ball hard (measured in different ways, but combined for 48% — average exit velocity, barrels per batted ball event, percentage of batted balls hit greater than 95 MPH, maximum exit velocity and average distance)
- Don’t hit the ball soft (5% — percentage of batted balls poorly topped)
For reasons that will become clearer in the “testing” section below, let’s call this metric: Prediction of wOBA Attempt (POWA), preferably pronounced as “POW-uh.”
2019 POWA Leaderboard: Top & Bottom 15%
More importantly, who ranks well by POWA? Here’s a leaderboard with the top and bottom 15% POWA hitters from 2019 (minimum 30 PA):
As you might expect, that Mike Trout fellow tops the 2019 leaderboard — he’s pretty good, isn’t he? As you might also expect, he’s surrounded by a who’s who of elite sluggers: Joey Gallo, Christian Yelich, Aaron Judge, Cody Bellinger, Giancarlo Stanton, Josh Donaldson, Juan Soto and many more. At the very least, POWA passes our initial sanity check.
Testing POWA
Does it pass slightly more rigorous testing, however? Should we be using POWA to evaluate hitters? Or is it part of the “problem,” introducing additional noise and complexity into a world already exploding with new metrics? Let’s test and find out — specifically, we’ll test its predictiveness and season-to-season reliability (often referred to as “stickiness”).
Using the same testing sample (2015-2019 hitter data from Statcast, minimum 500 PA, n = 359 season pairs), here’s POWA’s predictiveness:
Without context, it’s hard to judge. Testing within the same sample, let’s compare POWA to wOBA and expected wOBA (xwOBA):
Predict wOBA_n+1 | |
Metric_n | r2 |
wOBA | 0.304 |
POWA | 0.270 |
xwOBA | 0.248 |
While not quite as predictive of wOBA as wOBA itself — hence the “A” in POWA, for “attempt” — POWA did test as nearly 10% more predictive of future wOBA than the more widely used xwOBA! It tested even better against other “expected” stats, such as expected batting average (xBA) or expected slugging percentage (xSLG).
(To be fair, xwOBA and other “expected” stats weren’t necessarily designed to be predictive. According to Tom Tango, Senior Database Architect of Stats for MLB Advanced Media, xwOBA was designed to be descriptive. I surmise the results would be different — and likely better — if they were intended to be predictive.)
Still, you might be disappointed to see that you could use plain old wOBA to better predict future performance. I hear you. However, despite being slightly less predictive than wOBA, season-to-season stickiness is where POWA really shines:
With an r-squared exceeding 0.66, POWA is far stickier season-to-season than both wOBA and xwOBA:
Predict Itself_n+1 | |
Metric_n | r2 |
POWA | 0.661 |
xwOBA | 0.448 |
wOBA | 0.304 |
In other words, if a hitter displays POWA skills in one season, they are much likelier to display those skills the following season — at least relative to skills captured by wOBA and xwOBA. Given POWA largely includes only raw skills — strikeouts, walks, exit velocities, etc. — rather than outcomes like wOBA, that shouldn’t be particularly surprising.
It may be a statistical oversimplification, but here’s another way to think about it: approximately 66% of a hitter’s POWA score can be explained by the previous season’s POWA score. By contrast, a hitter’s wOBA in one season is explained by only 30% of their previous season’s wOBA — simply put, wOBA is much more volatile season-to-season.
To recap, POWA is more predictive of future wOBA than xwOBA and more than two times stickier than wOBA. Not bad!
Finding POWA
So how should we be using POWA? It’s great that it identifies Mike Trout and Christian Yelich as elite hitters, but then again, we didn’t need any fancy math equations to figure that out. Instead, POWA might help us identify under-the-radar hitters going later in Fantasy drafts but with similarly compelling raw hitting talents. Stay tuned for more, when we’ll identify just who some of those late hitting targets might be … find the POWA.
Appendix: Methodology
The methodology used to calculate POWA is eerily similar to ACES — that is, it relies on the use of z-scores across each incorporated statistic:
- Z-scores are calculated across each statistic (e.g., strikeout rate, walk rate, average exit velocity, etc.) using the average and standard deviation from the full 2015-2019 player universe (minimum 500 PA)
- Z-scores for each statistic are weighted by the determined weights mentioned above in the piece:
- Plate Discipline — 47%
- Strikeout Rate (K%) — 27%
- Walk Rate (BB%) — 20%
- Contact Quality — 53%
- Average Exit Velocity — 15%
- Barrels per Batted Ball Event — 14%
- % of Batted Balls Hit 95+ MPH — 10%
- Maximum Exit Velocity — 6%
- % of Batted Balls Poorly Topped — 5%
- Average Distance — 3%
- The weighted z-scores are then summed, resulting in a hitter’s POWA score
- Percentiles are calculated within each season (e.g., Mike Trout’s 100th percentile POWA rank in 2019 is relative to 2019 hitters only)
- Plate Discipline — 47%
Download Our Free News & Alerts Mobile App
Like what you see? Download our updated fantasy baseball app for iPhone and Android with 24x7 player news, injury alerts, sleepers, prospects & more. All free!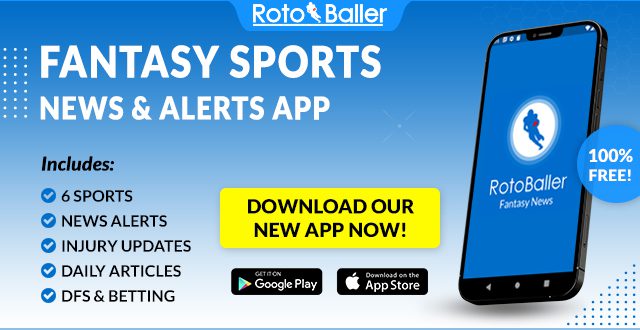
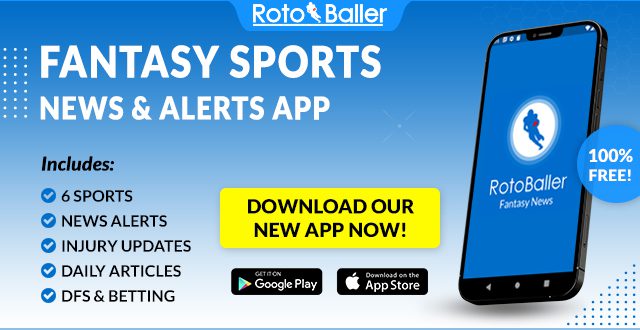