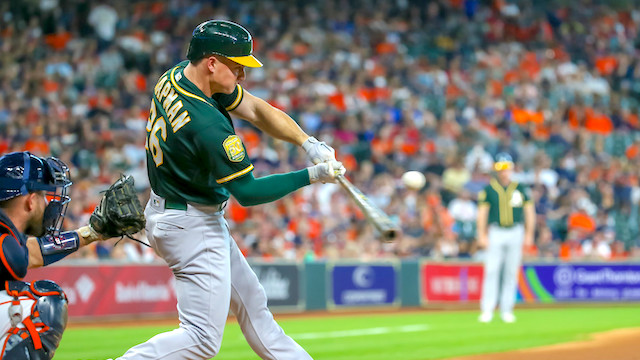
Barrels are the keeper of life’s finer things. Beer, pickles, monkeys, the list is endless.
Barrels don’t just hold fermented vegetables and unruly simians these days, barrels may also hold the key to identifying the game’s best hitters and struggling hitters who may be due for brighter days.
In my mission to find just how important Statcast is to surface stats, and to find the “best” Statcast metric to judge hitters, I’ve landed firmly on barrel rate, and I’m going to explain why it’s the best and why you should be paying close attention to it when evaluating hitters.
Featured Promo: Get any full-season MLB and DFS Premium Pass for 50% off. Exclusive access to our Team Sync platform, Premium articles, daily Matchup Rating projections, 15 lineup tools, DFS cheat sheets, Research Stations, Lineup Optimizers and much more! Sign Up Now!
What is a Barrel?
Webster’s dictionary defines a barrel as “a cylindrical container bulging out in the middle, traditionally made of wooden staves with metal hoops around them.” That's interesting, but that definition only provides moderate insight into the contemporary advanced baseball metric. In baseball, a barrel is essentially the ideal contact a batter can make. Like a Reese’s cup, a barrel has the perfect ratio of peanut butter and chocolate, with exit velocity acting as the peanut butter and launch angle acting as the chocolate. A barrel is the best possible way a batter can strike a ball.
Barrels are calculated on a slope depending on how hard the ball is struck. MLB’s example states “A ball traveling 99 mph always earns 'Barreled' status when struck between 25-31 degrees. Add one more mph -- to reach 100 -- and the range grows another three degrees, to 24-33.” Going back to our candy example, if we add more peanut butter (exit velocity) we’ve got to also increase our chocolate (launch angle) or we no longer have a peanut butter cup. We’ve probably still got something tasty, like a single, a double, or a Reese’s Easter Egg, but it’s not the ideal ratio.
Admittedly, when barrels were first introduced by MLB I didn’t pay them as much attention. Like many baseball nerds, I was enthralled by Statcast when it came out, and am still enthralled to this day, but barrels felt a bit abstract for me compared to the other metrics. That changed this offseason, when I decided it was time to stop merely trusting Statcast and actually testing the metrics against real outcomes, and what I found pointed me straight to barrels.
Since Statcast debuted I just kind of accepted the importance of these metrics. Logically, it makes sense. We’ve known harder hit balls are better, flyballs lead to home runs, and that Yadier Molina is really really slow long before Statcast quantified this for us. And the individual examples seemed to confirm the importance of Statcast metrics. To demonstrate this, let’s play a game. Below are the Statcast metrics for two centerfielders. One belongs to an MVP winner, and the other belongs to a player who’s never received an MVP vote. Can you guess which is which?
If you guessed the bottom entry belongs to the MVP, congratulations, you’ve identified Mike Trout. If you guessed the top entry (Billy Hamilton) belongs to the MVP, I’d encourage you to keep reading and check out all of the baseball articles on this site, as you’ve got lots more research to do before draft day.
Sure, those were two extreme examples, but the point stands. Individuals helped foster my trust for Statcast metrics. I mean, why question Statcast? It’s clearly doing its job if it identifies Mike Trout as a strong hitter and Billy Hamilton as a weak hitter. But there a lot of guys in between Mike Trout and Billy Hamilton, and I wanted to know how much these metrics meant for them too.
Testing Statcast Metrics
I began by downloading the 2020 qualified hitter leaderboards from the bible (AKA fangraphs.com), generating a custom report that would give me both Statcast data and surface stats for every qualified hitter. Then, I ran a function that produces the coefficient between any two metrics and tested the Statcast correlation against two outcomes: Batting Average (AVG), and Isolated Power (ISO).
Batting average was of course my first choice, as it can be influenced by so many outside factors such as ballpark, fielder position, sprint speed, etc. that I wanted to know if we could boil down any Statcast metric to help us predict AVG. ISO was my second choice because it is the best rate stat at capturing a hitter’s power, and I suspected that Statcast metrics would have a stronger correlation to a hitter’s power than a hitter’s batting average because power is more in a player’s control compared to batting average.
(The next few paragraphs reference a few mathematical concepts. All you need to know is that a coefficient of r >0 indicates a positive correlation, and a coefficient of r <0 indicates negative correlation. The closer those values are to 1 or -1 respectively, the stronger the relationship. A value of 1 is a perfect positive correlation, and -1 indicates perfect negative correlation)
When I ran the calculations I was pleasantly unsurprised to find that most of the metrics I believed to have an influence on a player’s power numbers actually did have influence. This was much less true for batting average, but that will be addressed in a moment. When it came to power, one metric stood out above the rest, and that was barrel rate. Most of the other metrics had weak to moderate correlation, but when I correlated barrel rate to ISO for 2020 hitters I got a coefficient of r = 0.79, indicating a strong correlation between the two.
With 2020 giving us a wonky season, I widened my data set to include every qualified hitter between 2015-2020, and was able to reproduce the results with essentially the same outcome (r = 0.82). Because including only qualified hitters may lead to survivor bias (hitters that produce better outcomes will be more likely earn enough plate appearances to qualify, skewing it towards the best hitters) I widened my data set one more time to include every hitter with at least 150 PAs between 2015-2020, which included a total of 714 unique players. And? Numbers don’t lie, as I reproduced the results and got r = 0.81. There is a strong relationship between barrel rate and ISO, stronger than any other metrics by a good measure. I ran a linear regression and got p<0.0001, which means we'd get the same outcome in 9,999 of 10,000 MLB seasons.
As I said before, I didn’t find as strong of correlation with other Statcast metrics to ISO, and found weak or no correlation with Statcast metrics to batting average. See the chart below (the value is the coefficient between the metric and the outcome):
ISO | AVG | |
Barrel Rate | 0.812767 | 0.029294 |
Max Exit Velo | 0.522001 | 0.109035 |
Average Exit Velo | 0.613493 | 0.174133 |
Hard Hit Rate | 0.662493 | 0.156718 |
Line Drive Rate | -0.10563 | 0.345165 |
Flyball Rate | 0.543557 | -0.25638 |
Average Launch Angle | 0.45844 | -0.19038 |
K Rate | 0.240587 | -0.48534 |
BB Rate | 0.322577 | -0.07739 |
Groundball Rate | -0.49552 | 0.094206 |
And here's a graph to help visualize the relationship:
Not much scatter to that plot, eh? It symbolizes a near-perfect linear relationship between ISO and barrel rate. These results make sense, because if a barrel is indeed ideal contact, then extra-base hits or home runs are the ideal outcome. Barrels have the privilege of precision not afforded to other raw metrics like exit velocity or launch angle. It was designed by humans to identify the best-struck balls, and those humans did a pretty good job.
Even after getting confirmation that Statcast and barrels specifically are great for analyzing hitters, a few questions remain. How much variance is there in a player’s barrel rate? How do we predict if a hitter will see a spike or drop in barrel rate? And, most importantly, how can we use this to win at fantasy baseball?
How Much Does Barrel Rate Vary by Player?
First, let’s tackle how much variance an individual player might have in their barrel rate. I calculated the average barrel rate of all 714 players in my data set and compared it against the players MIN and MAX barrel rate for their career. This should help give us an idea of much swing we could expect in barrel rate on a year-to-year basis. I’d like you to look at the table below:
It’s stained maple. I’m thinking of getting one for my kitchen, but hit me up on Twitter or Reddit if you have other suggestions. Now that that’s out of the way, check out this table below:
Name | '15 | '16 | '17 | '18 | '19 | '20 | Grand Total | Seasons w/ Data | Avg. Rate | Max. Rate | Max. Avg. | Min. | Avg. Min. |
A.J. Ellis | 5.5% | 2.8% | 3.4% | 4.2% | 15.9% | 4 | 3.98% | 5.50% | 1.52% | 2.80% | 1.17% | ||
A.J. Pierzynski | 2.9% | 0.9% | 3.8% | 2 | 1.90% | 2.90% | 1.00% | 0.90% | 1.00% | ||||
A.J. Pollock | 4.5% | 5.4% | 9.7% | 7.6% | 10.5% | 37.7% | 5 | 7.54% | 10.50% | 2.96% | 4.50% | 3.04% | |
Aaron Altherr | 2.0% | 3.1% | 9.7% | 9.7% | 24.5% | 4 | 6.13% | 9.70% | 3.57% | 2.00% | 4.12% | ||
Aaron Hicks | 2.8% | 3.8% | 7.1% | 8.5% | 7.9% | 6.9% | 37.0% | 6 | 6.17% | 8.50% | 2.33% | 2.80% | 3.36% |
Aaron Hill | 1.9% | 1.2% | 3.1% | 2 | 1.55% | 1.90% | 0.35% | 1.20% | 0.35% | ||||
Aaron Judge | 24.9% | 15.4% | 19.7% | 60% | 3 | 20.00% | 24.90% | 4.90% | 15.40% | 4.60% | |||
Abraham Almonte | 5.9% | 5.6% | 4.7% | 7.0% | 23.2% | 4 | 5.80% | 7.00% | 1.20% | 4.70% | 1.10% | ||
Adalberto Mondesi | 10.9% | 7.2% | 7.3% | 25.4% | 3 | 8.47% | 10.90% | 2.43% | 7.20% | 1.26% | |||
Adam Duvall | 11.1% | 8.4% | 9.7% | 13.9% | 43.1% | 4 | 10.78% | 13.90% | 3.12% | 8.40% | 2.37% | ||
Adam Eaton | 2.9% | 2.7% | 3.1% | 3.6% | 4.6% | 16.9% | 5 | 3.38% | 4.60% | 1.22% | 2.70% | 0.68% | |
Adam Engel | 3.6% | 2.3% | 4.7% | 10.6% | 3 | 3.53% | 4.70% | 1.16% | 2.30% | 1.23% | |||
Adam Frazier | 1.7% | 2% | 3% | 1.9% | 4% | 12.6% | 5 | 2.52% | 4.00% | 1.48% | 1.70% | 0.82% | |
Adam Haseley | 3.1% | 3.1% | 1 | 3.10% | 3.10% | 0.00% | 3.10% | 0.00% | |||||
Adam Jones | 8.7% | 7% | 5.7% | 4.9% | 5.7% | 32.0% | 5 | 6.40% | 8.70% | 2.30% | 4.90% | 1.50% | |
Adam LaRoche | 7.4% | 7.4% | 1 | 7.40% | 7.40% | 0.00% | 7.40% | 0.00% | |||||
Adam Lind | 6.2% | 8% | 7.1% | 21.3% | 3 | 7.10% | 8.00% | 0.90% | 6.20% | 0.90% | |||
Adam Rosales | 10.8% | 3% | 13.8% | 2 | 6.90% | 10.80% | 3.90% | 3.00% | 3.90% | ||||
Addison Russell | 4.6% | 8.8% | 5.0% | 2.2% | 6.3% | 26.9% | 5 | 5.38% | 8.80% | 3.42% | 2.20% | 3.18% | |
Adeiny Hechavarria | 1% | 0.7% | 2.6% | 1.6% | 4.5% | 10.4% | 5 | 2.08% | 4.50% | 2.42% | 0.70% | 1.38% | |
Adonis Garcia | 7% | 4.5% | 3.3% | 14.8% | 3 | 4.93% | 7.00% | 2.06% | 3.30% | 1.63% | |||
Adrian Beltre | 4.9% | 7.9% | 4.4% | 6.1% | 23.3% | 4 | 5.83% | 7.90% | 2.07% | 4.40% | 1.42% | ||
Adrian Gonzalez | 7.7% | 3.9% | 3.6% | 5.8% | 21.0% | 4 | 5.25% | 7.70% | 2.45% | 3.60% | 1.65% | ||
Albert Almora Jr. | 3.2% | 1.1% | 3.9% | 8.2% | 3 | 2.73% | 3.90% | 1.16% | 1.10% | 1.63% | |||
Albert Pujols | 7.1% | 8.8% | 4.8% | 5.7% | 5.3% | 5.5% | 37.2% | 6 | 6.20% | 8.80% | 2.60% | 4.80% | 1.40% |
Because the entire table has too many entries, I sampled a few names to give you an idea of my methodology. Mostly, players tend to stick around their average barrel rate every year. The mean difference between a player’s average barrel rate and max barrel rate (removing players with only one season of data) was 2.03%, and the difference was even smaller on the minimum side at just 1.83%. Only 29 of the 714 players deviated more than 5% higher than their average, and only 114 of the 714 players deviated 3% or higher than their average.
The results were even more extreme on the other side, as only 6 of the 714 players deviated 5% or more below their average barrel rate, and only 84 of the 714 deviated 3% or more below their average. The average barrel rate of the group was 5.89%, so a 3-5% swing would be pretty drastic.
So, we know barrel rate, like many metrics, can remain pretty predictable over a player’s career, but does anything help us predict a future improvement or reduction in barrel rate?
I tested the metrics available in my data set and found that outside of average and max exit velocity (they’re components of barrels, obviously they correlate), the strongest corollary was actually strikeout rate. Specifically, high strikeout rates. See the chart below (values represent coefficient between the two variables listed):
Barrel Rate | |
Line Drive Rate | -0.08628 |
Flyball Rate | 0.448431 |
Groundball Rate | -0.40917 |
K Rate | 0.5057 |
BB Rate | 0.388879 |
Average Launch Angle | 0.37576 |
Max Exit Velo | 0.617153 |
Average Exit Velo | 0.720367 |
I ran a linear regression and the results weren’t nearly as strong as barrel rate to power, and in fact, this relationship does not pass the scientific standard for statistical significance. As I analyzed some of the highest barrel rate hitters (Joey Gallo, Aaron Judge, Giancarlo Stanton) I realized that the top was populated with strikeout kings. Perhaps this means barrels are best achieved by selling out for power. Or perhaps only the strongest players can consistently achieve high barrel rates, and the strongest players happen to go down on strikes a lot. The ball needs to be smoked for a barrel, after all, with a 98 MPH exit velocity being the minimum exit velocity to achieve a barrel.
Average exit velocity only has a weak correlation with a high strikeout rate, and I don’t think a player would necessarily improve his barrel rate by raising his strikeout rate. A player with a barrel rate above 20% is usually on the end of a few extremes, and it seems more likely that the extreme outliers mentioned earlier carry too much of an influence on the group when it comes to strikeouts.
So, we can’t really use any traditional statistics to predict whether a player can improve his barrel rate, and players don’t tend to drastically increase or decrease their barrel rates on a yearly basis. Then how do we identify breakout candidates? I think it might be helpful to do a few case studies on fantasy-relevant players to try and reverse engineer their spike in barrel rates.
Corey Seager, Los Angeles Dodgers
7.3% 2019 barrel rate-15.8% 2020 barrel rate
Corey Seager enjoyed a spectacular 2020, posting a monster .943 OPS and .278 ISO in 232 PA. His success was buoyed by a stark jump in barrel rate, as Seager maintained an impressive 15.8% barrel rate, a rate 6.45% higher than his career average and an 8.5% jump from 2019. It’s safe to say the catalyst behind Seager’s leap in barrel rate was not an increase in strikeouts, as young shortstop cut his strikeout rate to 15.9%, the second-lowest of his career. Interestingly, Seager did post a career-worst 13.4% SwStr rate, and the fact that he whiffed so much while maintaining a low strikeout rate speaks to one of the more important factors here, which is aggression.
Seager became more aggressive at the plate in 2020, increasing his swing rate by 4% and his zone swing rate by 5%, though his overall contact rate of 76% is in line with his career average. That coupled with an increase in average exit velocity to a blistering 93.3 MPH helped Seager improve his barrel rate. What most likely happened in Seager’s case is health paired with a change in approach. We already knew Seager was talented, but injuries caused his overall numbers and Statcast metrics to dip. Perhaps the best way to identify potential barrel rate risers would be to look at players with past success who’ve tumbled due to injury or other outside factors, but still possess the ability to light up a Statcast sheet. If Seager can keep this up he'll find himself an MVP contender in no time.
Matt Chapman, Oakland Athletics
12.1% 2019 barrel rate - 18% 2020 barrel rate
Chapman took an already strong power game to the next level in 2020, posting a career-high .303 ISO in 152 PA. That power can be attributed to an 18% barrel rate, roughly triple the league average. Unlike Corey Seager, Chapman did experience a sharp increase in strikeout rate. Chapman went down on strikeouts 35.5% of the time in 2020, a staggering 13.6% increase from 2019. It was a year of extremes indeed for Chapman, who also increased his flyball rate to 50.6% and increased his average launch angle to 24.6-degrees. Chapman’s case gives more credence to the high strikeouts=high barrel rate hypothesis, because Chapman took an extreme, and like Seager, a more aggressive approach at the plate.
While this approach will undoubtedly lead to more power, it may not be the best approach for Chapman from a real baseball perspective. He profiled very similarly to Miguel Sano last season, which is definitely an above-average bat, but Chapman seems capable of a more balanced approach based on past seasons. Another point Chapman’s case touches is the correlation between batting average and barrel rate, which is practically non-existent. A player like this might have a really high barrel rate because he struggles to make contact, leaving fewer batted ball events per plate appearance, but when he does the contact is exceptional. Chapman presents an intriguing option at NFBC ADP of 114. He isn’t a player I’ve explicitly targeted this draft season, but he’s an interesting player to watch in 2021 and is probably worth grabbing a share or two to see where he goes.
Ultimately, it seems like too many factors can influence a spike like both Seager and Chapman experienced, making it hard to predict. Trying to identify players who might experience a spike in barrel rate is probably overly complex and unnecessary, as there are easier ways to gain value from this information.
Applying the Knowledge
Now that we know definitively the positive correlation between barrels and power production, you’re probably wondering how this information can be used to help you win at fantasy baseball. It’s one thing to deep dive into the numbers for the heck of it, but there’s got to be something applicable here, right?
Check in for part two in order to find out which players could see a power surge in 2021 based on barrel-rate analysis!
Download Our Free News & Alerts Mobile App
Like what you see? Download our updated fantasy baseball app for iPhone and Android with 24x7 player news, injury alerts, sleepers, prospects & more. All free!
More Fantasy Baseball Advice